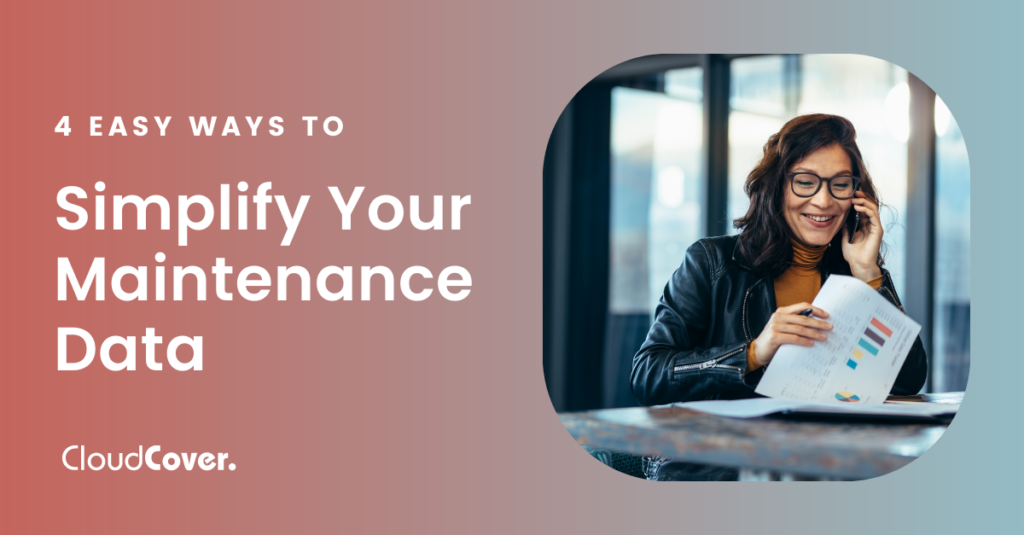
4 Easy Ways to Simplify Your Maintenance Data
- 5 Minute Read
- Maintenance Management
In this post:
It’s no surprise that your maintenance data grows every day. According to IDC, more than 59 zettabytes (ZB) of data will be created, captured, copied, and consumed globally this year (IDC’s Global DataSphere Forecast).
While that stat is intimidating, there is hope for anyone trying make sense of large, complex collections of maintenance data. Below are four proven methods to consider.
Perspective is Key
Remember those quizzes that show you a close-up picture of an everyday object, asking you to guess what the object is? Your guess on what you’re looking at is confined to just that very small perspective. How often did you find yourself unable to discern what you’re looking at? How often are you surprised when the identity of the object is revealed?
Perspective can be everything when it comes to data. For instance, asking a group of 3 people a simple yes or no question doesn’t mean you’ll get the same results when you expand your survey to a group of, say, 30 people. In fact, adding more people may make your results the complete opposite of what a smaller survey originally seemed to suggest.
This is because the more people you add to your survey, the more variables you will likely encounter, and the more things you will need to consider when trying to understand why your results look the way they do. This is why two out of three can yield much different results than twenty out of thirty, or thirty out of one hundred. Simply zooming out widens the picture and changes your perspective and observations on what you see. It widens the scale and adds new context to consider.
For something as complex as maintenance data, where the picture includes not just a total sum to consider but also a variety of geographies, types of server, storage, and networking equipment, as well as vendors and SLA’s, keeping an understanding on the perspective from which you are viewing the data becomes key.
Always stop and take a good look at the parameters around any set of data. Before drawing a conclusion on what the picture is, give yourself the chance to zoom in and out and see how that changes your understanding on what you think you’re observing.
Regardless of what you’re looking at, having a clear understanding on your perspective is important. It allows you to understand why the data you are seeing looks the way it does – and perhaps most importantly – what it means for your business.
Group Data Accordingly
Let’s go back to the survey example but let’s say you’ve decided to ask even more people – an even hundred. More data may seem like it’s only going to cause more complexities, but again, perspective is key. Instead of looking at a group of people (or a collection of equipment) as one large data set, find a way to organize them into smaller groups within your overall count.
When you start to group your equipment by certain criteria, say geography or age, you start to see that the results become more homogenous along specific variables within each group. Now predicting becomes much easier.
No matter how large your data set, if you can group it according to similarities and differences, you can also control it much more easily. With data grouped into subsets you can reach that same two-thirds result, by simply preselecting certain respondents more likely to answer according to demographics. Better control on your data means you can create better outcomes for yourself and your business.
To Find a Solution, Allow Yourself Room for Complex Problems
Grouping data is helpful but it’s also important to acknowledge that there can be many complexities when looking at certain types of data. For this kind of data, top-line summaries don’t usually tell the whole story. The data around Service Level Agreement (SLA) delays is a good example of data that requires a deeper examination.
When trying optimize a solution for this type of data, you must be willing to see it as a complex problem. This means not just stopping at what appears to be the first solution but taking the time to test and review that solution to see if it still holds under each analysis. This approach can take more time at the beginning, but it ensures you don’t end up wasting time and resources implementing the wrong solution.
CloudCover encountered a recent example where we found taking the time to really examine what at first glance, seemed to be a simple SLA failure.

The chart above graphs the amount of time the average cases sat in a particular status. Each of these cases are from the last year of services. Recently we began using a custom-built AI to help manage this partner as we worked to better understand their problems. The result can be seen through a month by month decrease in overall delays.
The chart identifies different cycles in a case. At first glance, you might be tempted to think that delays are mostly caused by the diagnosis taking too long. But that’s not what’s happening.
After evaluation, we found that “diagnosis” was not the primary cause of delays. The “part order,” “scheduling a technician,” and “customer caused delays” took up the majority of the case lifecycle. Below is the math used to evaluate the sums of status. Using data science to understand where the problem really resided meant we could build a solution that actually addressed solving for it.

At CloudCover, we are not in the business of simply solving problems. We use Data Science to prove that our hypothesis is correct about the problem, first. Once we thoroughly understand the problem, we move to solve it.
Details are always important but getting too involved in singular details (i.e., focusing only on the timetable for diagnosing and none of the other parts) sometimes leads to the old “can’t see the forest for the trees” problem. By allowing for complex problems, you can ensure you’re seeing the whole forest, rather than just a single tree.
Find the Right Smart Tools to Do the Heavy Lifting
Unfortunately getting data boiled down into these clear recommendations isn’t always easy. Too many companies are still relying on Excel sheets to track their warranty and service maintenance data and asking their sales teams to update them “as needed.” This strategy leads to human error, forgotten updates by an already busy sales team, and no consistency around any of the customer information.
There are maintenance companies selling “smart” software solutions that can help you get a better grasp on your data but not all solutions are created equal.
Questions to Ask When Evaluating Any Maintenance Management Solution
-
- Do they have a dedicated developer team in-house should problems arise?
- What is their integration process?
- Can they connect with your current technology investments?
- Do they have a dedicated data science team ensuring their tool’s algorithms are firing correctly as variables are added or changed? You need to feel confident in any dashboard view.
Most importantly, certain features, like artificial intelligence, need to be more than just buzzwords. AI should not be static – ask them what improvements they have in the pipeline. Ask them for a specific example of how their AI helped a customer. If the company doesn’t have readily available answers to these questions, it may be worth expanding your search to different vendors’ offerings.
Understanding your maintenance data is key to growing your business. Want to learn more? Check out our newest report. There’s Money in Your Data: Revenue Growth Opportunities for Channel Partners.
