- Services
Maintenance and Services
Explore Our Support
- Products
Products and Features
Explore Key Features
- Partners
- Industries
- Resources
Resources
Learn and Explore
- Log In
- Get Started
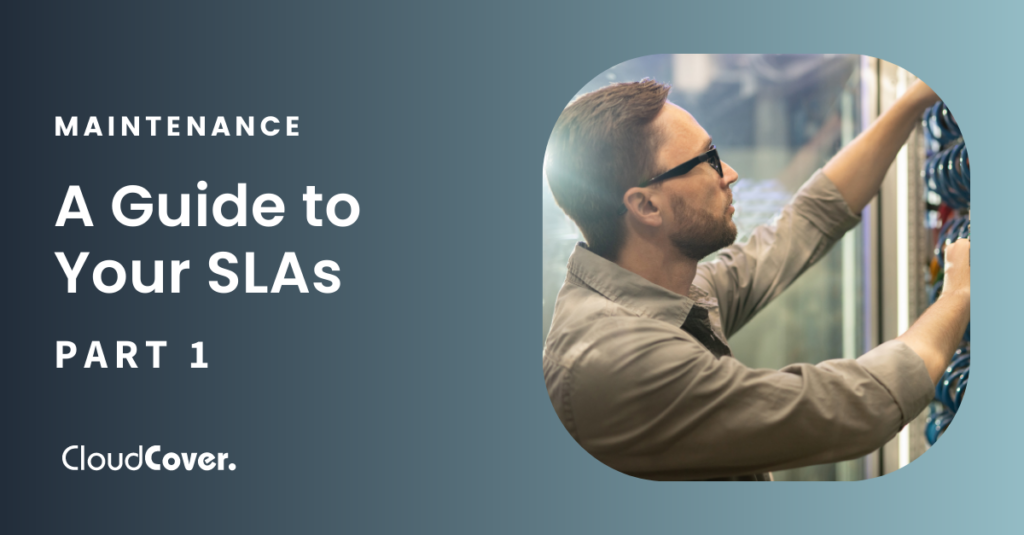
A Guide to Your SLA’s | Part 1
- 5 Minute Read
- SLAs
In this post:
The maintenance management ecosystem is a tangled web of acronyms and jargon, from downtime to uptime to Mean Time Between Failures (MTBF). Even those of us fluent with this terminology know there is a lack of consistency in usage among different sources. This is especially true for terms like service-level agreements (SLAs) and artificial intelligence (AI).
To help make sense of this, our director of marketing, Tracey Carl, spoke with our director of data science, Aaron Sorensen, who is not only a subject matter authority on all things maintenance management but also a sharp thinker who can skillfully break down difficult concepts. Aaron has been a great internal resource for us here at CloudCover, and we’re happy to be able to share his insights with a larger audience.
Our first interview covers how SLAs are typically defined and measured and how AI is helping deliver more efficient service. We have also provided definitions of key terms and some additional context about maintenance management.
What are service-level agreements (SLAs) and what do they generally cover?
In the context of maintenance services, a service-level agreement (SLA) is the summary of a contract between an original equipment manufacturer (OEM), Reseller, or third-party maintenance (TPM) provider and their customers that details the specific services to be provided and defines the minimum standards (including reliability of equipment and responsiveness to service requests) that the provider is obligated to meet.
Aaron clarified:
“When [we] say “summary of contract,” what [we] mean is we’re selling service delivery and we’re defining what that service delivery is going to do within a certain amount of time through an SLA, meaning a common SLA of 7x24x4. What that means is you can log a ticket seven days a week, twenty-four hours a day. So any time you have a ticket or you need to log a ticket, you can. We will acknowledge it and we’re going to do something in four hours. That’s what that means. “
The 7x24x4 framing for SLAs is very common, but the definition of “4” is often ambiguous and can have multiple interpretations:
“The big question behind the SLA is what is that four hours? The OEMs [original equipment manufacturers] typically mean we’re going to try to get parts there and engineering there in four hours. TPMs sometimes mean that they’re going to try to get parts and engineering there in four hours.”
[…]
“The OEM can clearly tell you, yes, we can deliver for our service because we know every component that’s inside that machine and we’ll stock them where a TPM may not know all of those components inside the machine. So having these conversations with TPMs is really important. It’s important for your company. It’s important for us to have these conversations, and it’s important for us to communicate exactly what we mean by an SLA.”
How is artificial intelligence (AI) and machine learning (ML) changing SLAs?
Machine learning (ML) is a subset of artificial intelligence that involves identifying patterns and associations within large and often complex datasets. ML, along with neural networks and deep learning, has been especially useful in IT maintenance management as it can be used to predict the probability of equipment failure, allowing TPMs to customize their inventories and engineering requirements and ultimately identify and approach potential delays before they happen.
Aaron explained how this ultimately helps clarify how response times will work for companies using CloudCover’s intelligent support:
“We now have the same tool sets that the OEMs have to predict what’s going to fail, what component levels are needed, which parts are going to need to be stocked, and where we have all of those tools and those capabilities, whether or not your team is using those tool sets. We are using those tool sets so we can be really clear about the SLA. That to us is 7x24x4. SLA means that we’re going to manage any type of case that comes in. We’re going to acknowledge [the case] within 30 minutes—the average time is actually about seven minutes for us. It’s again because we’re using artificial intelligence, we’re going to have a part and an engineer on-site in four hours, if not sooner. For every single case that comes up. So we’re actually building our support using AI so that we can deliver those SLAs so that I can be clear to you in a meeting when you ask, ‘What’s what is this SLA? What does it mean to you? How do you process this SLA?'”
Artificial intelligence is also useful in helping Channel providers guarantee more efficient service at lower costs:
“[With artificial intelligence] we’re building on a model of operational business that allows us to manage inventories in a much smarter way. We’re streamlining it. What we’re doing is risk management or risk profile in every single asset and we’re predicting what’s going to fail. And we’re absolutely dead-on on our predictions. [What we can do now is] take thousands of assets and see these are the only parts that we think we’re going to need in these cells.”
“What typically happens when you’re managing this type of model using AI is that the question isn’t ‘what’s going to fail?’ The question becomes, I’ve got a really low probability of failure for these components. There’s a three percent [chance of] failure. What are we going to do? We’re not going to stock those parts, but how are we going to deliver service if that [component] fails? And so the question gets turned upside down, with a company that’s using AI, and it becomes ‘what can we do to manage the cases or the potentials out there that are so low we’re not going to stock from?’ ‘How will we manage those if they come up?'”
“And that’s an interesting conversation that takes place at an operational level because we’ve never had those conversations before. The last 20 years, we’ve been doing this the same way. And now all of a sudden, we’re looking at things from the opposite side, which really delivers incredible service for companies. We’re selling great service, but the other thing this does is it drops the cost dramatically. I don’t have to charge as much when [the] model that I’m using to build out service delivery is really lean. And so I don’t have to charge companies as much.”
What sort of metrics should be considered when crafting an SLA?
SLAs not only define the level of service but also specify the metrics by which service is measured and adhered to—and the remedies or penalties that should occur should agreed-upon service levels not be achieved.
With that in mind, it’s important for companies to think carefully about these metrics when drawing up a service-level agreement. Aaron identifies several variables to consider, specifically with regard to how “diagnosis” is defined:
“When I say we’re going to provide four-hour parts and four-hour engineers, I of course don’t mean I’m going to provide a four-hour part before we’ve diagnosed the failure, right? [The clock] should start after the diagnosis. And that’s where a lot of [maintenance providers] get fuzzy. They end up saying, ‘Well, it could take us twenty-four hours to diagnose the problem. It could take us two days to diagnose the problem.’ [So they finally] diagnose it. Goodness, they were able to get a four-hour part on site after they diagnosed it because they waited to diagnose the component failure. That happens an awful lot in the industry.
“The question for you is what you bought when you bought the contract. Did you buy next-business-day service or did you buy [immediate service and action]? The reality is most companies will know that they don’t need a hard drive in four hours, and so they put up with that kind of stuff. The point that I’m making with companies is you don’t have to put up with that stuff. The reality is that I can deliver to you four-hour components, for anything that fails as long as I measure correctly and we charge the right amounts. And so the question is really, when do I begin diagnosing this. [With] 70 percent of all the cases that come in I can diagnose within a minute. In fact, our artificial intelligence is already diagnosing those things within a minute. So it’s the more difficult cases that we’re taking some time to diagnose, but it’s not taking 24 hours.”
IT maintenance support is often grouped into different tiers of service, within level one basically capturing help desk assistance, two being more in-depth technical support, and three being direct assistance from a product and service support specialist. Aaron states that difficult cases should be handled by level three technicians who can quickly diagnose the problem and thus quickly facilitate a hardware replacement or other solution. As such, TPM customers shouldn’t have to wait for 24 hours to receive a formal diagnosis, or have to wait even longer than that to have a replacement part shipped.
How is AI facilitating better customer service?
In addition to diagnostic functionality, AI can support providers through chatbots and other customer interface applications. Aaron explains how CloudCover is able to use AI to decrease response time:
“My goal in using AI is to increase the frequency of when we respond. So we’re looking to respond right away to a customer’s email or a customer’s request for a ticket. And the reason why that’s important is I have a greater chance of reaching that customer if I reply back right away. If it takes me 25 minutes to reply, they may have already gone on to some other problem, they’ve got more pressing issues to deal with. So I want to be able to respond to that client right away so I can get the information that I need to continue the case. And so we’re seeing an incredible advantage of immediate response, automated responses, AI responses that allows us to manage cases much faster than we’ve previously seen through manual processes.”
In part two of the interview, Tracey and Aaron will discuss the flexibility of SLAs, the importance of relationships between TPMs and OEMs, and how service is changing due to advances in AI technology (stay tuned for that link here).
Take a look at CloudCover
Gartner-recognized CloudCover is completely unique to the marketplace and offers incredible value and control of your maintenance environment. If you’d like to learn more about the CloudCover Model and see our platform in action, click below.